AI algorithms: (many) opportunities and (a few) threats
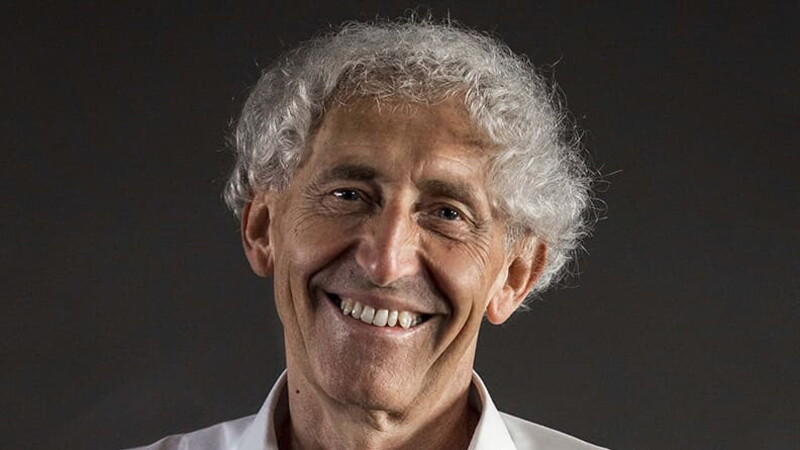
Mathematical models, Big Data (gigantic amounts of complex and heterogeneous data) and sophisticated artificial intelligence (AI) algorithms enable the construction of the hyperreality of the metaverse. In this parallel world, which can be reconstructed with great accuracy and realism, we can take advantage of extraordinary opportunities, such as visiting museums remotely, being driven by self-driving cars, even building digital twins of our own heart or brain. In doing so, we will have to resign ourselves to the presence of increasingly intrusive algorithms that can invade the most intimate spaces of our lives and reveal in advance (to us and to others: employers, insurance companies, political parties) our characteristics, weaknesses, preferences. But let us proceed in order.
A world of data
It was the US technology giant Cisco that identified the “Zettabyte Era” when global IP traffic first reached 1.2 zettabytes in 2016 (1 zettabyte = 1021 bytes = 1 trillion gigabytes, the equivalent of 36 million years of high-definition video). Data is incessantly being generated by satellites, sensors, CT or MRI images for clinical diagnosis and, of course, social media. Suffice it to say that more than 3 billion individuals now have access to the internet and every minute an estimated 300 hours of new videos are uploaded to YouTube, 350,000 tweets are produced on Twitter, 4,200,000 posts are made on Facebook, 1,700,000 photos are posted on Instagram, 110,000 calls are made on Skype, etc. On average, over the last 30 years, the data generated has quadrupled every three years or so.
This enormous rate of growth in data – accompanied by the spread of cloud computing, which provides access to great computing power at low cost, and the overarching development of artificial intelligence (AI) and machine learning (ML) algorithms in particular – on the one hand make the entire knowledge of the universe available “free of charge” to the individual and on the other hand allow Big Tech (such as Amazon, Apple, Microsoft, Google, Facebook...) to implement increasingly sophisticated algorithms thanks to the personalised data that each of us, consciously or unconsciously, provides.
Ever-improving performance
ML algorithms are able to automatically improve their performance through experience (i.e. through exposure to data). One of the main tools behind the success of machine learning is Artificial Neural Networks (ANN), complex mathematical systems that emulate the behaviour of human decision-making processes.
Thanks to ANNs, ML algorithms can provide answers without being explicitly programmed to address a given question, learning to do so autonomously on the basis of the available data. As a result, the computers on which they are implemented learn automatically and improve their learning capacity through ANN training made possible by ever new data.
The fields of application are virtually infinite: from automation to artificial vision (with application to autonomous driving), from voice recognition to written text recognition (think automatic translators), from text mining to data analytics, to name but a few.
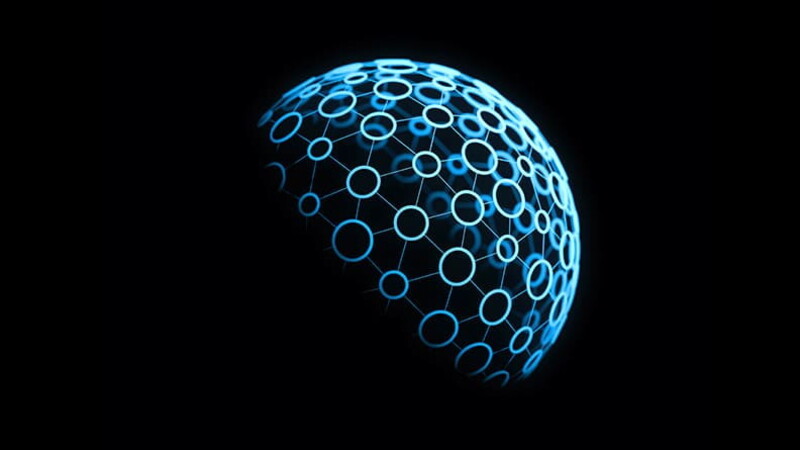
Two-way continuous dialogue
The new frontier is that of the digital twin, i.e. sets of virtual information constructs that mimic the structure, context and behaviour of an individual (or of a physical or technological process), dynamically updated thanks to the data derived from its physical twin throughout its life cycle and to informed decisions that generate value. A characteristic element of the digital twin is the two-way, continuous dialogue with the physical entity represented: on the one hand, the digital twin provides information to actively monitor and control the physical twin, on the other hand, the information generated by the real twin feeds the simulation algorithms of the digital twin.
Efficiently managing the immense amount of data flowing from physical assets to their virtual replicas and extracting knowledge from it are crucial aspects for the success of the digital twin paradigm. Enabling technologies for the emergence of digital twins are in fact the Internet of Things (IoT), cloud computing and Big Data. The widespread diffusion of these technologies and the increasingly lower cost of computing and storage resources are among the reasons for the recent spread of digital twins in industrial contexts.
Other contexts in which the use of digital twins is well established are the manufacturing industry, transport industry and the smart cities sector.
New possibilities
The digital twin paradigm, which was conceived and developed in the industrial sphere (first by NASA in the 1960s when it built replicas of its spacecraft to study their operation and possible malfunction on the ground), is increasingly being adopted in the health sector as well. Health expenditure is expanding rapidly (7 per cent growth each year), a rate that would lead it to exceed the entire GDP of Europe by 2070. It is therefore crucial to research new technologies to improve prevention and treatment. The continuous monitoring of people through wearable devices, integrated with ANNs, generates what has already been dubbed the IoH (Internet of Health). The development of digital twins of specific patients (also called human avatars) could revolutionise the healthcare industry, providing real-time guidance for prevention, diagnosis and treatment in a totally personalised manner. By resorting to these algorithms of “hyper-reality”, or augmented reality, we are in fact constructing a parallel, virtual reality, but no less “real” and effective in its ability to describe various physical processes.
We might be led to conclude that we are opening the door to many realities, but in essence we are “only” representing reality, with all its extraordinary difficulties, accurately and with predictive potential. Going beyond the present, simulating the future. Thanks to the availability of Big Data, mathematical models and AI algorithms. After all, this has already been done for decades with weather forecasts based on mathematical models.
While this is excellent news (in a few years, each of us will have a digital twin with which we can produce valuable diagnostic information), it also reveals one of the “threatening” aspects of artificial intelligence algorithms: the increasing intrusion into our lives. When algorithms make it possible to predict the onset and evolution of certain diseases to which one might be susceptible, one may wonder whether everyone is really ready to know about such predictions. And there is another aspect, at least just as critical. Simulations and early diagnosis may be of interest to our employers or medical insurance companies. Revealing our physical and psychological weaknesses, laying bare our desires, our sporting or political passions, our preferences as consumers or as readers. “Orienting” machine-learning algorithms to convey information to us with a strong bias, casting social or religious movements in a good or bad light, or some of the candidates in the upcoming elections, through blatant violations of fact checking.
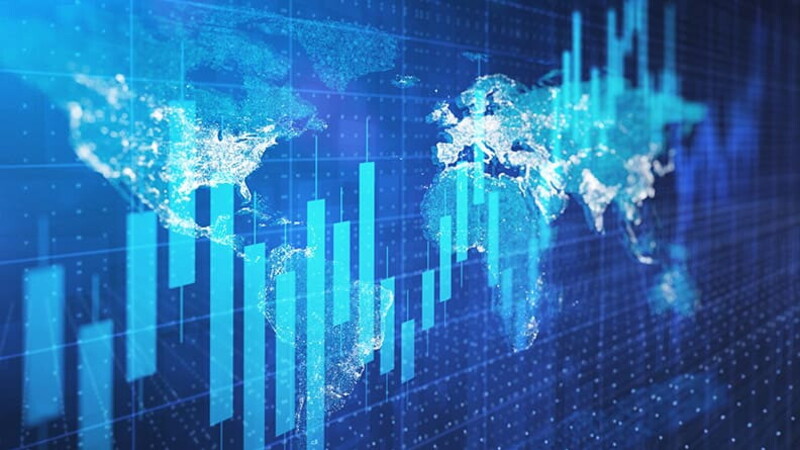
The data divide
More generally, and not just limited to digital twins, Big Data collected by powerful institutions or private companies is starting to raise doubts and social concerns. The digital divide between those who can not only access data, but also use it is widening, leading from a state of digital divide to a state of data divide. Big Data and AI algorithms enable a distributed cognitive system, which generates instances of accountability. Many individuals, groups and institutions end up sharing responsibility for the conceptual interpretation and social outcomes of specific uses of data.
A key challenge for the governance of Big Data is to find mechanisms for allocating responsibilities in this complex network, so that erroneous and unjustified decisions – as well as outright fraudulent, unethical, abusive, discriminatory or misleading actions – can be detected, corrected and appropriately sanctioned. The great opportunities offered by information and communication technologies entail a huge intellectual responsibility concerning their understanding and proper exploitation.
The need for algorithmic transparency
From a sociological point of view, the concern is that machine-learning algorithms may end up knowing us better than we know ourselves. The dreaded risk is that our employers or rulers may demand to know what their employees or citizens really want. If, for example, our governments have access to these algorithms and know our ideas and tastes, they will be able to intervene in our lives in the name of our good. Of course, algorithms capable of knowing ourselves to perfection can also come in handy, for example by showing us which choices could make us happier or healthier, which professional opportunities are best suited to us, which production or marketing strategies can increase our company's profits. The real issue is therefore to prevent these knowledge tools from remaining exclusively in the hands of others (and not ours).
The alternative to erecting shields to protect our privacy is that of algorithmic transparency, i.e. the claim that the structure, wills and decisions hidden in each algorithm are clear and explicit. One could demand that those who process our data to create knowledge about ourselves are legally obliged to return that knowledge to us. In this regard, someone applied the slogan “nothing about us without us” to the age of artificial intelligence. Difficult, however, to imagine that this could be accepted by Big Tech without a decisive transnational political initiative.